Avoid Complexity in Limiting Risk
Picture Credit: Olivier ROUX || Simplicity almost always beats complexity.
I’m not a fan of EIAs. I’m not a fan of variable annuities, unless they’re really simple with rock-bottom expenses and no surrender charges. I’m not a fan of ETNs. I hate structured notes. I’m also not a fan of ETFs that are filled with derivatives.
Ten years ago, I wrote a piece called The Good ETF. It is still as valid now as before, along with its companion piece The Good ETF, Part 2 (sort of). And for Commodity ETFs, there was: Fusion Solution: The Stable Value Fund Guide to Commodity ETF Management. If you are rolling futures in an ETF, it had better be done like a short bond ladder.
You can add in the pieces that I wrote before and when the short volatility ETFs imploded. What did it say in The Good ETF?
Good ETFs are:
* Small compared to the pool that they fish in
* Follow broad themes
* Do not rely on irreplicable assets
* Storable, they do not require a ?roll? or some replication strategy.
* not affected by unexpected credit events.
* Liquid in terms of what they represent, and liquid it what they hold.The last one is a good summary.? There are many ETFs that are Closed-end funds in disguise.? An ETF with liquid assets, following a theme that many will want to follow will never disappear, and will have a price that tracks its NAV.
The Good ETF
But tonight I have another complex investment to avoid, and a simple one to embrace. First the avoid…
There was a piece at Bloomberg Businessweek called ETFs With Downside Protection? It?s Complicated. These are called defined outcome ETFs. Basically they are a bundle of equity options that cut your losses, while limiting your gains on a given equity index. (Also, you don’t get dividend income, and have to pay manager fees.) In-between the cap on gains, and where losses kick in, your returns should move 1:1 with the index. The same will be true with losses after the first N% get eaten — below N% losses, you begin taking losses.
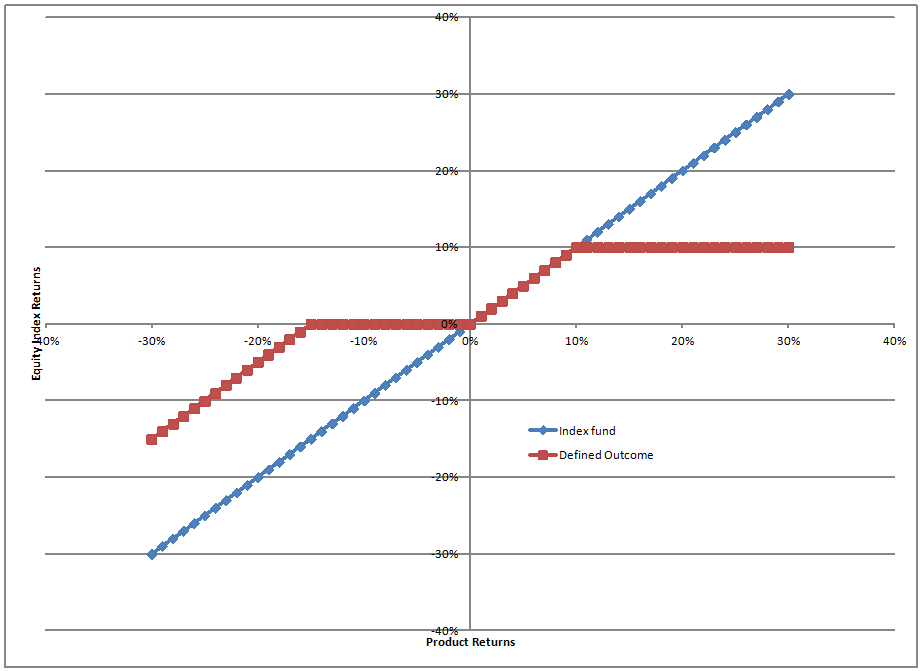
I wanted to keep the illustration simple. This hypothetical defined outcome ETF caps gains at 10%, and absorbs the first 15% of losses. This example assumes no fees, which would likely be lower on the index fund. This example assumes no dividends, which would get paid to you in the index fund, but not on the defined outcome ETF.
Defined outcome ETFs purchase and sell tailored options that are backed by the central counterparty the Options Clearing Corporation — a very strong, stable institution. Credit risk still exists, but if the OCC goes down, many things will be in trouble. The options exist for one year, after which gains are paid to and losses absorbed by ETF shareholders. The ETF then resets to start another year following the same strategy with slightly different levels because the relative amounts of the cap and the loss buffering rely on where equity volatility is for a given index at the start of the year.
Unlike an index fund, your gains cannot grow tax-deferred, though if you have gains, you can roll them over into the next year.
I’ve read the offering documents, including the sections on risk. My main argument with the product is that you give up too much upside for the downside protection. The really big up years are the places where you make your money. There aren’t so many “average” years. The protection on the downside is something, but in big down years it could be cold comfort.
The second part is the loss of dividends and paying higher fees. Using the S&P 500 as a proxy, a 2% dividend lost and a 0.5% added fee adds up to quite a cost.
There are implementation risks and credit risks but these risks are small. I ran a medium-sized EIA options book for a little more than a year. This is not rocket science. The investor who is comfortable with options could create this on his own. They list more risks in the offering documents, but they are small as well. What gets me are the costs, and the upside/downside tradeoff.
A Better, if Maligned Investment
Recently Bank of America declared ?the end of the 60-40? standard portfolio. I think this was foolish, and maligns one of the best strategies around — the balanced fund.
Yes, interest rates are low. Yields on some stocks are higher than the yields on the Barclays’ Aggregate [bond] Index. But if you only bought those bonds, you would have a rather unbalanced portfolio from a sector standpoint — heavy on utilities and financials. The Barclays’ Aggregate still outyields the S&P 500, if not by much, like 0.8%/yr.
The real reason that you hold bonds and cash equivalents is not the income; it is risk reduction. I’m assuming no one is thinking of buying the TLT ( 20+ Year Treas Bond Ishares ETF), which is more of a speculator’s vehicle, but something more like AGG ( US Aggregate Bond Ishares Core ETF), which yields 0.3% more, but the overall volatility is a lot less.
With AGG, fixed income claims of high investment grade entities will make it through a deflationary crisis. In an inflationary situation like the 70s, the bonds are short enough that over a five year period, you should make money, just not in real terms.
It’s good to think long term, and have a mix of fixed and variable claims. The bonds (fixed claims) lower your volatility so that you don’t get scared out of your stocks (variable claims) in a serious downdraft.
The models I have run have returns max out in an 80/20 balanced fund, and the trade-off of risk for return is pretty good down to a 60/40 balanced fund. In my personal investing, I have always been between 80/20 and 60/40.
As it is, if you are looking the likely returns on the S&P 500 over the next ten years, it’s about the same return available on a A3/A- corporate bond, but with a lot more volatility.
Thus the need for bonds. In a bad scenario, stocks will fall more than bonds, and the balanced fund will buy stocks using proceeds of the bonds that have fallen less to buy stocks more cheaply. And if the stock market rises further, the balanced fund will sell stocks and use the proceeds to bank the gains by buying bonds that will offer future risk reduction, and some income.
As such, consider the humble balanced fund as a long-term investment vehicle that is simple and enduring, even when rates are low. And avoid complexity in your investment dealings. It is almost never rewarded.